Visual Learning and Embodied Agents in Simulation Environments
ECCV 2018 Workshop, Munich, Germany
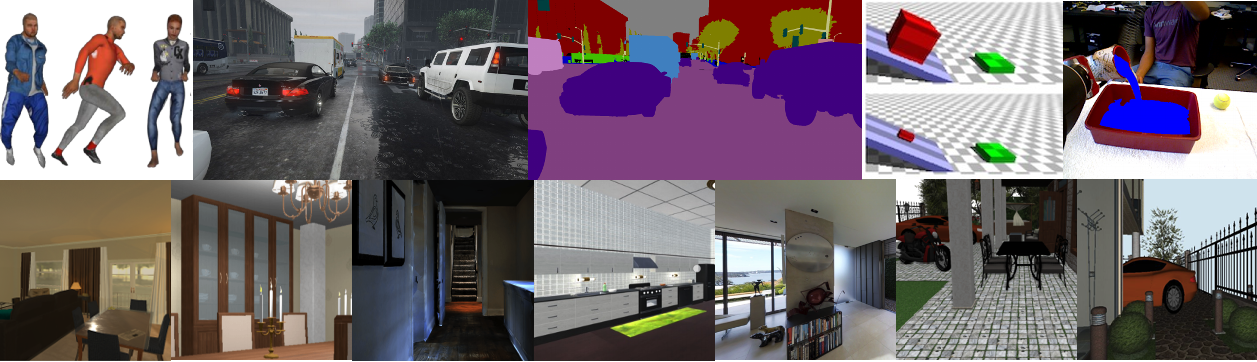
Image credit: [2, 28, 12, 11, 15-21, 26]
Introduction
Simulation environments are having a profound impact on computer vision and artificial intelligence (AI) research. Synthetic environments can be used to generate unlimited cheap, labeled data for training data-hungry visual learning algorithms for perception tasks such as 3D pose estimation [1, 2], object detection and recognition [3, 4], semantic segmentation [5], 3D reconstruction [6-9], intuitive physics modeling [10-13] and text localization [14]. In addition, visually-realistic simulation environments designed for embodied agents [15-21] have reignited interest in high-level AI tasks such as visual navigation [22, 23], natural language instruction following [20, 24, 25] and embodied question answering [26, 27]. This workshop will bring together researchers from computer vision, machine learning, natural language processing and robotics to examine the challenges and opportunities in this rapidly developing area - using simulation environments to develop intelligent embodied agents and other vision-based systems.
Call for Papers and Demos
We invite high-quality paper submissions, optionally with a live demo. Accepted papers will be presented during joint poster/demo sessions, with exceptional submissions selected for spotlight oral presentation.
Submissions must indicate if a poster-and-demo or a poster-only slot is requested. If a demo is requested, a short description of the demo and any equipment requirements must be provided. Note that demos should provide workshop participants with an opportunity to interact with simulation environments, algorithms, and agents in order to better understand the strengths and limitations of current work. Non-interactive visual displays, e.g. video or slide presentations will be rejected as demos.
Submissions are divided into two tracks and dual submission of a paper to both is prohibited:
Visual Learning Track
Topics: We welcome work focused on the use of synthetic data in broad computer vision tasks including but not limited to 3D pose estimation, object recognition, object detection, semantic segmentation, text localization, single-image 3D reconstruction, indoor/outdoor scene understanding, single-image VQA, and intuitive physics. Paper topics may include but are not limited to:
- Use of synthetic data in visual learning tasks
- Novel computer vision tasks using synthetic data
- Learning synthetic data generation protocols
- Domain adaptation from synthetic data to the real world
Submission: All Visual Learning Track submissions will be handled electronically via the workshop CMT website. Submissions to the Visual Learning Track should be between 4 and 14 pages in the ECCV format, excluding references, acknowledgements, and supplementary materials. All the accepted submissions will be published separately from the main conference in the post-proceedings by default, though authors could indicate explicitly if they want to opt out the post-proceedings. Dual submission is allowed, but must be explicitly stated at the time of submission and will not be included in the post-proceedings. Reviewing will be double-blind. Each submission will be reviewed by at least three reviewers for originality, significance, clarity, soundness, relevance and technical contents. Papers that are not blind, or have the wrong format, or have either less than 4 pages or more than 14 pages (excluding references) will be rejected without review. Please contact vlease2018.visuallearning@gmail.com for any concerns.
Embodied Agents Track
Topics: We invite extended abstracts for work on embodied agents operating in simulation environments including reinforcement learning and approaches that use mapping and planning. Paper topics may include but are not limited to:
- Novel datasets / simulators / tasks for embodied agents
- Language-based command of embodied agents, including embodied question answering and / or dialog
- Photo-realistic simulations from reconstructed point clouds / 3D meshes
- Simulating interactions with objects, other agents, and environmental changes
- Domain adaptation for embodied agents
Submission: For the Embodied Agents Track, we encourage 6 page submissions excluding references, acknowledgements, and supplementary material. The submission should be in the ECCV format. Reviewing will be single blind. Accepted extended abstracts will be made publicly available as non-archival reports, allowing future submissions to archival conferences or journals. We also welcome published papers that are within the scope of the workshop (without re-formatting), including papers from the main ECCV conference. Please submit your Embodied Agents Track paper to the following address by the deadline: embodiedagents@gmail.com. Please mention in your email if your submission has already been accepted for publication (and the name of the conference).
Important Dates
Paper Submission Deadline (extended!) | |
Final Decisions | |
Workshop Date | September 9th, 2018 |
Schedule
- 08:45 AM : Welcome and Introduction
- 09:00 AM : Invited Talk: Peter Welinder
- Learning dexterity [slides]
- 09:25 AM : Invited Talk: Alan Yuille
- When Big Datasets are not Enough : The Need for Virtual Visual Worlds [slides]
- 09:50 AM : Invited Talk: Sanja Fidler
- 10:15 AM : Coffee + Posters/Demos (Both Tracks)
- 11:00 AM : Invited Talk: Boqing Gong
- Domain Adaptation and Transfer: All You Need to Use Simulation "for Real" [slides]
- 11:25 AM : Invited Talk: Lawson Wong
- Grounding Natural Language Instructions to Robot Behavior: A Goal-Directed View [slides]
- 11:50 AM : Poster Spotlight Presentations
- Visual Representations for Semantic Target Driven Navigation - Arsalan Mousavian et al.
- Answering Visual What-If Questions: From Actions to Predicted Scene Descriptions - Hector Basevi et al.
- Talk the Walk: Navigating New York City through Grounded Dialogue - Harm de Vries et al. [pdf]
- 12:05 PM : Environment Spotlight Presentations
- 12:30 PM : Lunch
- 01:30 PM : Invited Talk: Abhinav Gupta
- The Battle of (Slow) Real vs. (Faster) Fake : An Alternative View
- 01:55 PM : Invited Talk: Anton van den Hengel
- Problems with open set Vision and Language problems [slides]
- 02:20 PM : Invited Talk: Vladlen Koltun
- Learning to Navigate [slides]
- 02:45 PM : Coffee + Posters/Demos (Both Tracks)
- 03:30 PM : Invited Talk: Raia Hadsell
- Learning to Navigate ... at City Scale [slides]
- 03:55 PM : Invited Talk: Dhruv Batra
- A-STAR: Agents that See, Talk, Act and Reason [slides]
- 04:20 PM : Invited Talk: Jitendra Malik
- 04:45 PM : Panel Discussion (All Invited Speakers)
- 05:30 PM : Close
Accepted Papers
Visual Learning Track
- Modeling Camera Effects to Improve Visual Learning from Synthetic Data - Alexandra K Carlson, Katherine A Skinner, Matthew Johnson Roberson
- Adversarial Attacks Beyond the Image Space - Xiaohui Zeng, Chenxi Liu, Yu-Siang Wang, Weichao Qiu, Lingxi Xie, Yu-Wing Tai, Chi-Keung Tang, Alan Yuille
- Answering Visual What-If Questions: From Actions to Predicted Scene Descriptions - Hector Basevi, Misha Wagner, Rakshith Shetty, Wenbin Li, Mateusz Malinowski, Mario Fritz, Ales Leonardis
- Visual Representations for Semantic Target Driven Navigation - Arsalan Mousavian, Alexander Toshev, Marek Fiser, Jana Kosecka,, James Davidson
Embodied Agents Track
- Vision-and-Language Navigation: Interpreting visually-grounded navigation instructions in real environments - Peter Anderson, Qi Wu, Damien Teney, Jake Bruce, Mark Johnson, Niko Sunderhauf, Ian Reid, Stephen Gould, Anton van den Hengel [pdf]
- Grounding Natural Language Instructions to Semantic Goal Representations for Abstraction and Generalization - Dilip Arumugam*, Siddharth Karamcheti*, Nakul Gopalan, Edward C. Williams, Mina Rhee Lawson, L.S. Wong, Stefanie Tellex [pdf]
- Following High-level Navigation Instructions on a Simulated Quadcopter with Imitation Learning - Valts Blukis, Nataly Brukhim, Andrew Bennett, Ross A. Knepper, Yoav Artzi [pdf]
- Speaker-Follower Models for Vision-and-Language Navigation - Daniel Fried*, Ronghang Hu*, Volkan Cirik, Anna Rohrbach, Jacob Andreas, Louis-Philippe Morency, Taylor Berg-Kirkpatrick, Kate Saenko, Dan Klein**, Trevor Darrell** [pdf]
- Deep Reinforcement Learning of an Agent in a Modern 3D Video Game - Samuel Arzt, Gerhard Mitterlechner, Markus Tatzgern, and Thomas Stutz [pdf]
- Neural Modular Control for Embodied Question Answering - Abhishek Das, Georgia Gkioxari, Stefan Lee, Devi Parikh, Dhruv Batra [pdf]
- Building Generalizable Agents with a Realistic and Rich 3D Environment - Yi Wu, Yuxin Wu, Georgia Gkioxari, Yuandong Tian, Aviv Tamar, Stuart Russell [pdf]
- Learning a Semantic Prior for Guided Navigation - Yi Wu, Yuxin Wu, Georgia Gkioxari, Yuandong Tian [pdf]
- Look Before You Leap: Bridging Model-Free and Model-Based Reinforcement Learning for Planned-Ahead Vision-and-Language Navigation - Xin Wang*, Wenhan Xiong**, Hongmin Wang, William Yang Wang [pdf]
- Talk the Walk: Navigating New York City through Grounded Dialogue - Harm de Vries, Kurt Shuster, Dhruv Batra, Devi Parikh, Jason Weston & Douwe Kiela [pdf]
- MINOS: Multimodal Indoor Simulator for Navigation in Complex Environments - Manolis Savva, Angel X. Chang, Alexey Dosovitskiy, Thomas Funkhouser, Vladlen Koltun [pdf]
- Gibson Env: Real-World Perception for Embodied Agents - Fei Xia*, Amir R. Zamir*, Zhiyang He*, Alexander Sax, Jitendra Malik, Silvio Savarese [pdf]
Invited Speakers
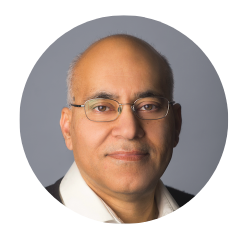
Jitendra Malik is the Arthur J. Chick Professor in the Department of Electrical Engineering and Computer Sciences at UC Berkeley. His research group has worked on many different topics in computer vision, computational modeling of human vision and computer graphics. Several well-known concepts and algorithms arose in this research, such as normalized cuts, high dynamic range imaging and R-CNN. He has mentored more than 50 PhD students and postdoctoral fellows. [Webpage]
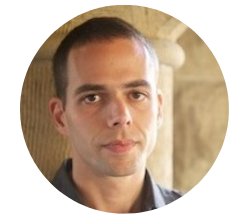
Vladlen Koltun is a Senior Principal Researcher and the director of the Intelligent Systems Lab at Intel. The lab is devoted to high-impact basic research on intelligent systems. Previously, he has been a Senior Research Scientist at Adobe Research and an Assistant Professor at Stanford where his theoretical research was recognized with the National Science Foundation (NSF) CAREER Award (2006) and the Sloan Research Fellowship (2007). [Webpage]
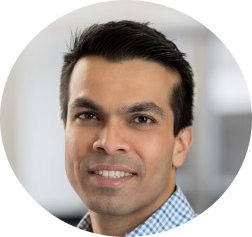
Dhruv Batra is an Assistant Professor in the School of Interactive Computing at Georgia Tech and a Research Scientist at Facebook AI Research (FAIR). His research interests lie at the intersection of machine learning, computer vision, natural language processing, and AI. He is a recipient of numerous awards including the Office of Naval Research (ONR) Young Investigator Program (YIP) award (2016), two Google Faculty Research Awards (2013, 2015) and the Amazon Academic Research award (2016). [Webpage]
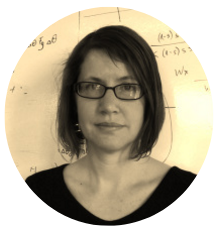
Raia Hadsell, a senior research scientist at DeepMind, has worked on deep learning and robotics problems for over 10 years. After completing a PhD with Yann LeCun at NYU, her research continued at Carnegie Mellon's Robotics Institute and SRI International, and in early 2014 she joined DeepMind in London to study artificial general intelligence. Her current research focuses on the challenge of continual learning for AI agents and robotic systems. [Webpage]
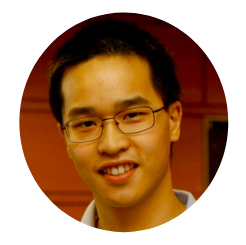
Lawson Wong is a postdoctoral fellow at Brown University, working with Stefanie Tellex. He completed his Ph.D. in 2016 at the Massachusetts Institute of Technology, advised by Leslie Pack Kaelbling and Tomás Lozano-Pérez. His current research focuses on acquiring, representing, and estimating knowledge about the world that an autonomous robot may find useful. He was awarded a AAAI Robotics Student Fellowship in 2015. [Webpage]
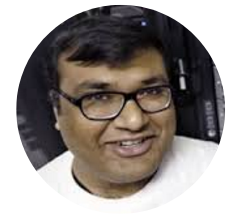
Abhinav Gupta is an Assistant Professor in the Robotics Institute at Carnegie Mellon University (CMU). Prior to this, he was a post-doctoral fellow at CMU working with Alyosha Efros and Martial Hebert. His research interests include developing representations of the visual world, linking language and vision, and the relationships between objects and actions. He is a recipient of the PAMI Young Researcher award, the Bosch Young Faculty Fellowship and a Google Faculty Research Award. [Webpage]
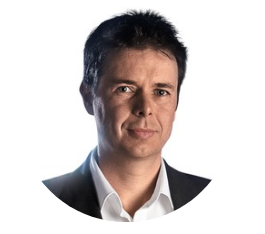
Anton van den Hengel is a Professor in the School of Computer Science at the University of Adelaide in Australia, the founding Director of the Australian Centre for Visual Technologies (ACVT), a Chief Investigator of the Australian Centre for Robotic Vision and a Program Leader in the Data 2 Decisions Cooperative Research Centre. He has won best paper at CVPR, published over 300 publications, had eight patents commercialized and founded two startups. [Webpage]
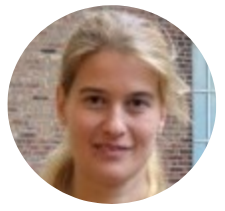
Sanja Fidler is an Assistant Professor at University of Toronto. Her main research interests are 2D and 3D object detection, particularly scalable multi-class detection, object segmentation and image labeling, and (3D) scene understanding. She is also interested in the interplay between language and vision. [Webpage]
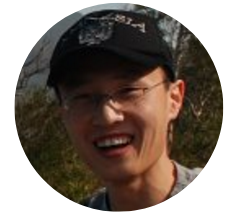
Boqing Gong is a Principal Researcher at Tencent AI Lab in Seattle, working on machine learning and computer vision. Before joining Tencent, he was a tenure-track Assistant Professor in University of Central Florida (UCF). He received a Ph.D. in Computer Science from the University of Southern California, where his work was partially supported by the Viterbi Fellowship. [Webpage]
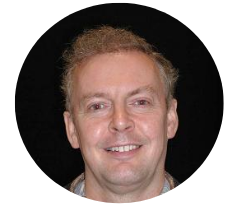
Alan Yuille is a Bloomberg Distinguished Professor of Cognitive Science and Computer Science at Johns Hopkins University. He directs the research group on Compositional Cognition, Vision, and Learning. He is affiliated with the Center for Brains, Minds and Machines, and the NSF Expedition in Computing, Visual Cortex On Silicon. His research interests include computational models of vision, mathematical models of cognition, medical image analysis, and artificial intelligence and neural networks. [Webpage]
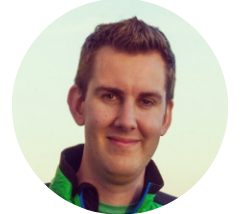
Peter Welinder is a research scientist at OpenAI. He works on topics ranging from deep reinforcement learning and computer vision to robotics software/hardware and simulation/rendering. Previously he founded and managed the Machine Learning Team at Dropbox. [Webpage]
Organizers
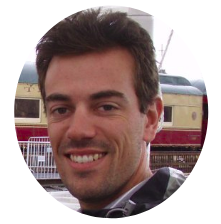
Georgia Tech
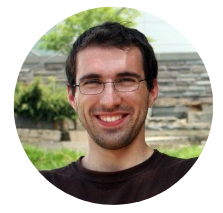
Facebook AI Research, Simon Fraser University
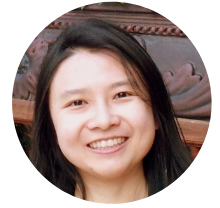
Eloquent Labs, Simon Fraser University
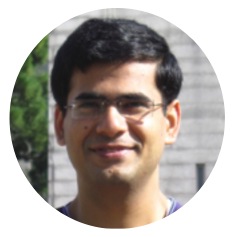
UC Berkeley
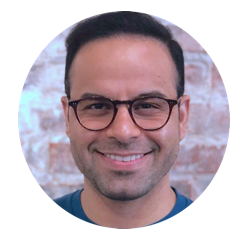
Stanford University, UC Berkeley
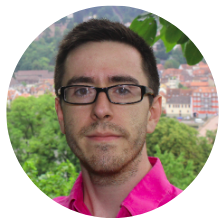
Georgia Tech
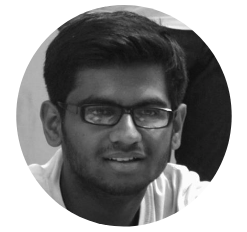
Georgia Tech
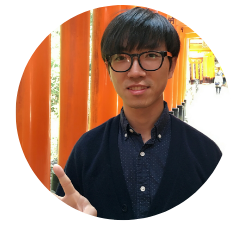
Stanford University
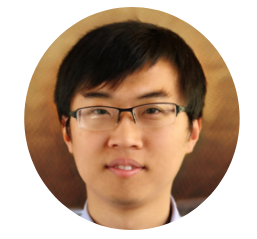
UC San Diego
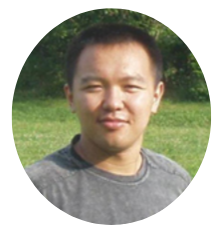
UT Austin
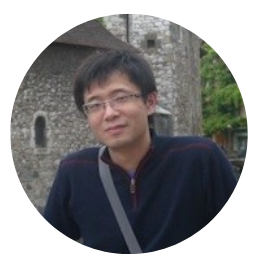
Shanghai Jiao Tong University
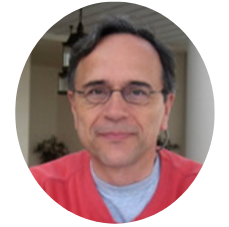
Stanford University
Acknowledgments
Thanks to visualdialog.org for the webpage format.
References
- Su, H., Qi, C.R., Li, Y., Guibas, L.J.: Render for cnn: Viewpoint estimation in images using cnns trained with rendered 3d model views. In: Proceedings of the IEEE International Conference on Computer Vision. (2015) 2686–2694
- Chen, W., Wang, H., Li, Y., Su, H., Wang, Z., Tu, C., Lischinski, D., Cohen-Or, D., Chen, B.: Synthesizing training images for boosting human 3d pose estimation. In: 3D Vision (3DV), 2016 Fourth International Conference on, IEEE (2016) 479–488
- Toshev, A., Makadia, A., Daniilidis, K.: Shape-based object recognition in videos using 3d synthetic object models. In: Computer Vision and Pattern Recognition, 2009. CVPR 2009. IEEE Conference on, IEEE (2009) 288–295
- Georgakis, G., Mousavian, A., Berg, A.C., Kosecka, J.: Synthesizing training data for object detection in indoor scenes. arXiv preprint arXiv:1702.07836 (2017)
- Ros, G., Sellart, L., Materzynska, J., Vazquez, D., Lopez, A.M.: The synthia dataset: A large collection of synthetic images for semantic segmentation of urban scenes. In: Proceedings of the IEEE Conference on Computer Vision and Pattern Recognition. (2016) 3234–3243
- Choy, C.B., Xu, D., Gwak, J., Chen, K., Savarese, S.: 3d-r2n2: A unified approach for single and multi-view 3d object reconstruction. In: European Conference on Computer Vision, Springer (2016) 628–644
- Fan, H., Su, H., Guibas, L.: A point set generation network for 3d object reconstruction from a single image. In: Conference on Computer Vision and Pattern Recognition (CVPR). Volume 38. (2017)
- Tatarchenko, M., Dosovitskiy, A., Brox, T.: Octree generating networks: Efficient convolutional architectures for high-resolution 3d outputs. CoRR, abs/1703.09438 (2017)
- Kar, A., Häne, C., Malik, J.: Learning a multi-view stereo machine. In: Advances in Neural Information Processing Systems. (2017) 364–375
- Byravan, A., Fox, D.: Se3-nets: Learning rigid body motion using deep neural networks. In: Robotics and Automation (ICRA), 2017 IEEE International Conference on, IEEE (2017) 173–180
- Schenck, C., Fox, D.: Reasoning about liquids via closed-loop simulation. arXiv preprint arXiv:1703.01656 (2017)
- Wu, J., Yildirim, I., Lim, J.J., Freeman, B., Tenenbaum, J.: Galileo: Perceiving physical object properties by integrating a physics engine with deep learning. In: Advances in neural information processing systems. (2015) 127–135
- Wu, J., Lu, E., Kohli, P., Freeman, B., Tenenbaum, J.: Learning to see physics via visual de-animation. In: Advances in Neural Information Processing Systems. (2017) 152–163
- Gupta, A., Vedaldi, A., Zisserman, A.: Synthetic data for text localisation in natural images. In: Proceedings of the IEEE Conference on Computer Vision and Pattern Recognition. (2016) 2315–2324
- Brodeur, S., Perez, E., Anand, A., Golemo, F., Celotti, L., Strub, F., Rouat, J., Larochelle, H., Courville, A.: HoME: A household multimodal environment. arXiv:1711.11017 (2017)
- Kolve, E., Mottaghi, R., Gordon, D., Zhu, Y., Gupta, A., Farhadi, A.: AI2-THOR: An interactive 3D environment for visual AI. arXiv:1712.05474 (2017)
- Wu, Y., Wu, Y., Gkioxari, G., Tian, Y.: Building generalizable agents with a realistic and rich 3D environment. arXiv:1801.02209 (2018)
- Yan, C., Misra, D., Bennnett, A., Walsman, A., Bisk, Y., Artzi, Y.: CHALET: Cornell house agent learning environment. arXiv:1801.07357 (2018)
- Savva, M., Chang, A.X., Dosovitskiy, A., Funkhouser, T., Koltun, V.: MINOS: Multimodal indoor simulator for navigation in complex environments. arXiv:1712.03931 (2017)
- Anderson, P., Wu, Q., Teney, D., Bruce, J., Johnson, M., Sünderhauf, N., Reid, I., Gould, S., van den Hengel, A.: Vision-and-Language Navigation: Interpreting visually-grounded navigation instructions in real environments. In: CVPR. (2018)
- Zamir, A.R., Xia, F., He, J., Sax, S., Malik, J., Savarese, S.: Gibson Env: Real-world perception for embodied agents. In: CVPR. (2018)
- Zhu, Y., Mottaghi, R., Kolve, E., Lim, J.J., Gupta, A., Fei-Fei, L., Farhadi, A.: Target-driven visual navigation in indoor scenes using deep reinforcement learning. In: ICRA. (2017)
- Gupta, S., Davidson, J., Levine, S., Sukthankar, R., Malik, J.: Cognitive mapping and planning for visual navigation. In: CVPR. (2017)
- Chaplot, D.S., Sathyendra, K.M., Pasumarthi, R.K., Rajagopal, D., Salakhutdinov, R.: Gated-attention architectures for task-oriented language grounding. In: AAAI. (2018)
- Misra, D.K., Langford, J., Artzi, Y.: Mapping instructions and visual observations to actions with reinforcement learning. In: EMNLP. (2017)
- Das, A., Datta, S., Gkioxari, G., Lee, S., Parikh, D., Batra, D.: Embodied Question Answering. In: CVPR. (2018)
- Gordon, D., Kembhavi, A., Rastegari, M., Redmon, J., Fox, D., Farhadi, A.: IQA: Visual question answering in interactive environments. In: CVPR. (2018)
- Richter, S., Vineet, V., Roth, S., Koltun, V.: Playing for Data: Ground Truth from Computer Games. In ECCV (2016).